Machine Learning for Fault Prediction in Manufacturing Industries
The manufacturing industry is no stranger to unexpected failures, leading to costly downtime, reduced productivity, and dissatisfied customers. However, the advent of machine learning has opened up new possibilities for proactive failure prediction, enabling manufacturers to mitigate risks, optimize maintenance schedules, and enhance overall efficiency, leading to better results. Today, we discuss how machine learning can be utilized for failure prediction in the manufacturing industry.
IMPORTANCE OF FAILURE PREDICTION IN MANUFACTURING
In an increasingly competitive marketplace, manufacturers face immense pressure to minimize operational disruptions and maintain consistent product quality. Failure prediction serves as a powerful tool to remain competitive in this rapidly changing landscape. By leveraging historical data and advanced machine learning algorithms, manufacturers can identify patterns and precursors to failures, enabling them to intervene before catastrophic breakdowns occur. Not only does this prevent costly downtime, but it also allows manufacturers to optimize maintenance strategies, reduce spare parts inventory, and enhance overall equipment effectiveness.
REAL LIFE EXAMPLES
GLOBAL ELECTRIC (GE)
GE is a global conglomerate that manufactures a wide range of products, including aircraft engines, power turbines, and medical equipment. The company has been using machine learning for failure prediction for several years, and has reported significant benefits. Bill Ruh, the CEO of GE Digital and GE’s Chief Digital Officer, emphasizes the role of machine learning in the successful implementation of digital twins. Digital twins are data-based replicas of industrial machines made by collecting real-time data from sensors embedded within the machine, capturing attributes like heat, vibration, and noise. The collected data is then organized and stored in the cloud, forming a model that replicates the machine’s performance. These replicas can then diagnose faults and predict maintenance needs, thereby reducing or eliminating unplanned downtime.
SIEMENS
Siemens provides predictive maintenance solutions to industries like manufacturing and energy. Hayat Kimya, a major diaper manufacturer, uses Siemens’ Predictive Services to enhance the efficiency and reliability of its drive systems. With machine learning, potential issues are identified in advance, enabling preventive action and reducing unplanned downtime. This approach improves efficiency and has environmental benefits by saving energy and reducing emissions.
ROLLS-ROYCE
Rolls-Royce is a British multinational aerospace company that manufactures aircraft engines, defense systems, and marine propulsion systems. The company has been using machine learning for failure prediction since 2016, and has reported that the technology has helped to improve the reliability of its engines.
GARANT
Garant is a Canadian manufacturer of outdoor hand tools. The company has been using machine learning for failure prediction since 2013, and has reported that the technology has helped to reduce warranty costs and improve product quality.
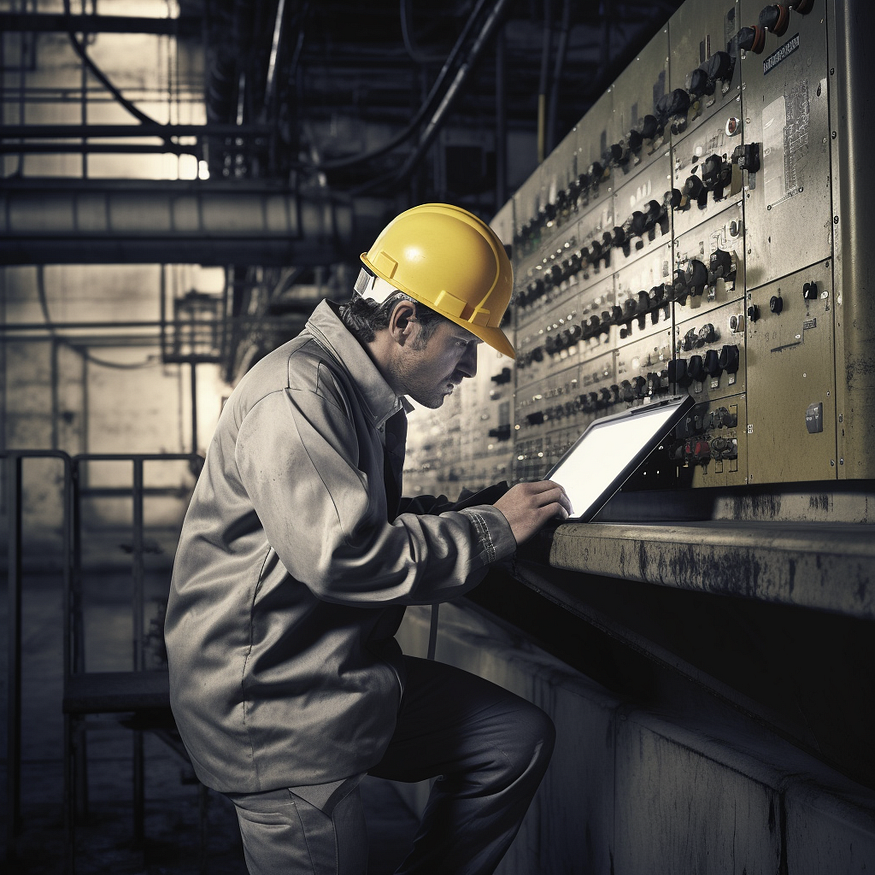
BENEFITS
- Proactive Maintenance: Machine learning enables manufacturers to shift from reactive to proactive maintenance strategies. By accurately predicting equipment failures, maintenance can be scheduled in advance, reducing costly unplanned downtime and optimizing resource allocation.
- Improved Equipment Efficiency: Machine learning algorithms can analyze operational data to identify inefficiencies in machinery and processes. By pinpointing areas for improvement, manufacturers can optimize equipment settings, reduce energy consumption, and enhance overall production efficiency.
- Enhanced Product Quality: By predicting potential failures, machine learning can identify issues that may impact product quality. Manufacturers can take corrective measures in advance, ensuring that defective products are minimized and customer satisfaction is maximized.
- Cost Savings: Preventing equipment failures and minimizing downtime not only saves on maintenance costs but also reduces the need for emergency repairs and replacement parts. Machine learning-driven failure prediction allows manufacturers to operate more cost-effectively and allocate resources efficiently.
KEY TIPS FOR EFFECTIVE UTILIZATION OF MACHINE LEARNING
- Data Availability: Obtaining relevant data for machine learning-based fault prediction can be a challenge.
- Data Quality and Standardization: Ensuring accurate and consistent data is crucial for reliable fault prediction with machine learning.
- Scalability: Scaling machine learning models for complex industrial systems requires careful consideration of resources.
- Model Training and Validation: Skilled expertise is needed to develop and fine-tune machine learning models for effective fault prediction.
- Integration with Existing Systems: Seamless integration with current software systems is essential when implementing machine learning for fault prediction.
- Cost and ROI: Assessing the cost implications and potential return on investment is necessary before adopting machine learning for fault prediction.
- Change Management and Adoption: Overcoming resistance to change and fostering a data-driven culture are key to successful adoption of machine learning in fault prediction.
- Continuous Model Updates and Maintenance: Regular updates and maintenance are critical for ensuring the effectiveness of machine learning models in fault prediction.
Conclusion
Machine learning is revolutionizing the manufacturing industry by enabling accurate failure prediction and proactive maintenance practices. The advantages are clear. By adopting failure prediction, companies can increase productivity, reduce expenses, and stay ahead of the competition. It’s an investment in your business’s success that will pay dividends for years to come. Don’t wait until it’s too late to start incorporating this invaluable tool into your manufacturing process. Take advantage of failure prediction technology and propel your business forward in today’s increasingly competitive marketplace.
At Ruffin Galactic, we specialize in leveraging data to drive business success. Our team of data scientists and machine learning experts can help you harness the power of your data to predict failures, optimize maintenance schedules, and enhance overall efficiency. We offer tailored solutions to meet your specific needs, helping you stay competitive in the marketplace. Don’t wait until it’s too late — visit www.ruffingalactic.com today and let us help you transform your manufacturing processes with the power of data and machine learning.
Discover how predictive quality analytics are revolutionizing the manufacturing industry in our article here.
Related Resources

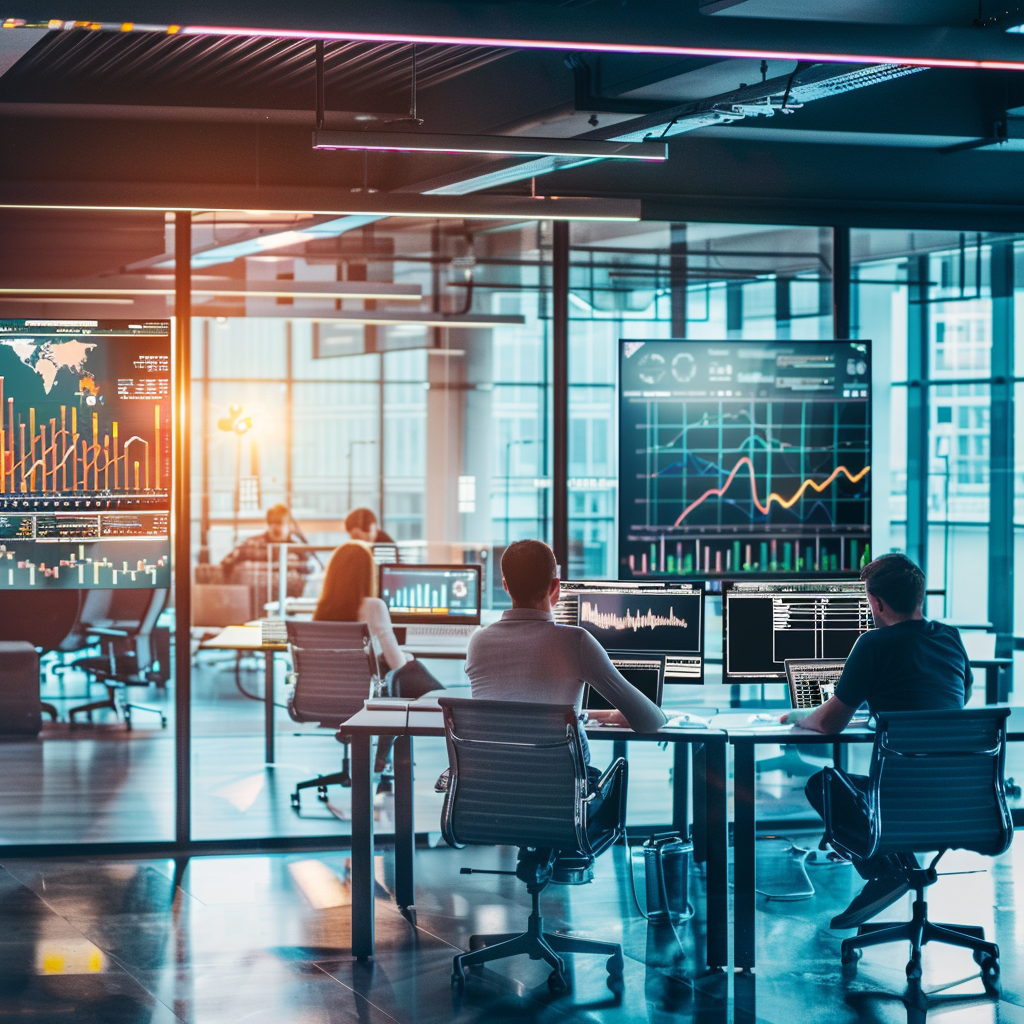
