Predictive Quality Analytics in Manufacturing
In the highly competitive manufacturing landscape, manufacturers constantly struggle to balance customer satisfaction with cost control. Increasing competition and customer demands, coupled with complex products and innovations, create challenges in ensuring defect-free products. The traditional approach to quality control often involved reactive measures, relying on inspections and post-production analysis. However, with technological advancements, predictive quality analytics has emerged as a game-changer in the manufacturing industry. By leveraging data-driven insights, predictive quality analytics empowers manufacturers to proactively identify and address quality issues before they arise, leading to enhanced efficiency, reduced costs, and improved customer satisfaction.
Typically, manufacturers ensure quality after production by checking products for flaws and errors. The issue with this approach is that it requires manual checking, which is time-consuming and occasionally inaccurate, and it checks for issues after they have occurred, rendering some products wasted. Predictive quality analytics offers an appealing alternative.
BENEFITS OF PREDICTIVE QUALITY ANALYTICS
Predictive quality analytics offers numerous benefits to manufacturers. It allows them to proactively identify potential issues, prioritize them based on severity, and investigate them thoroughly before they become major concerns. Manufacturers can reduce manual effort and enable targeted investigations into specific quality issues by automating and streamlining the investigation process. Continuous monitoring and analysis of quality data also help manufacturers quickly identify deviations or non-compliance issues, allowing them to respond promptly and take appropriate corrective actions to meet regulatory obligations. Through predictive quality analytics, manufacturers can generate risk-ranked safety alerts that prioritize the severity and urgency of quality issues, helping them efficiently allocate resources and manage cases by focusing on high-priority issues that require immediate attention. Predictive quality analytics also allows manufacturers to reduce costs related to warranty claims, product recalls, and fraudulent activities while improving brand reputation and enhancing customer satisfaction. By consistently delivering high-quality products, manufacturers build trust with customers and establish a positive brand image. Additionally, predictive quality analytics helps manufacturers to minimize waste and optimize processes by identifying areas of waste, quality variations, and inefficiencies, leading to reduced rework, improved resource utilization, and lower production costs, contributing to overall cost efficiency. Finally, predictive quality analytics facilitates collaboration across the supply chain by sharing quality data and insights with suppliers and partners, allowing manufacturers to work together to identify and address potential quality issues, strengthening relationships, improving supply chain transparency, and ensuring the delivery of high-quality outputs.
HOW IT WORKS?
All accessible external and internal data sources are prioritized, gathered, and correlated. Comprehensive data analysis is conducted at the analytics level, and predictive models are created using a number of different evaluation methods iteratively. These techniques can foresee both problems that manifest soon after purchase and those that could eventually result in a warranty claim, depending on the inputs that flow into the model.
At a very high level there are three steps:
- Data Collection
- Data Analysis
- Implementation
Step 1: Data Collection
The first step in predictive quality analytics is to gather data from various sources, including:
- Voice of the Customer (VoC): Gathering customer feedback through surveys, feedback forms, social media monitoring, and online reviews helps identify potential quality issues and prioritize improvement efforts.
- Sales Feedback: Insights from frontline salespeople, who interact directly with the product, provide valuable information on everyday issues, recurring problems, and emerging trends.
- IoT: Real-time data collected from connected systems, such as performance, usage, and diagnostic information, can help detect patterns, anomalies and predict quality issues early on.
Step 2: Data Analysis
After that, we need to do advanced analytics, including, but not limited to:
- Theme Discovery: Analyzing textual data to identify common topics or themes related to quality issues using text mining and sentiment analysis techniques.
- Anomaly Detection: Identifying unusual patterns or deviations from expected behavior in quality-related variables through statistical modeling or machine learning algorithms.
- Concept Extraction: Extracting key concepts or terms from unstructured data to gain a deeper understanding of quality-related factors.
- Text Clustering: Grouping similar documents or text based on content to efficiently categorize and analyze quality-related information.
- Probability Analysis: Assessing the likelihood of specific quality outcomes or events occurring based on historical data or relevant variables.
- Event History Analysis: Studying the time until the occurrence of quality events using statistical models to identify critical factors and develop preventive strategies.
Step 3: Implementation
The third and final step involves the implementation of solutions, such as:
- Prioritization Analysis: Analyze and prioritize quality issues based on their severity, impact on customer satisfaction, and potential costs. This analysis helps identify the most critical quality concerns that require immediate attention and allocation of resources.
- Risk-Ranked Alerts: Implement a system that generates risk-ranked alerts based on data analysis and predictive models. These alerts notify stakeholders about potential quality issues, their associated risks, and the urgency of action required. Risk-ranked alerts ensure that the focus is on addressing high-priority quality concerns.
Once the recommendation and solutions are implemented, it is essential to establish appropriate measures to address the identified quality issues. This can include process improvements, design modifications, supplier quality management, training programs, or any other necessary actions to mitigate risks and improve overall quality.
CONCLUSION
In essence, predictive quality analytics empowers manufacturers to transcend traditional quality control paradigms and embrace a proactive, data-driven approach. By leveraging the vast potential of data analytics, manufacturers can pave the way for improved operational efficiency, reduced costs, and enhanced customer satisfaction. Embracing this transformative technology is not merely a competitive advantage but a strategic imperative for manufacturers seeking to thrive in a rapidly changing world. To learn more about how your company can benefit from this technology, visit our website at http://www.ruffingalactic.com. With our cutting-edge solutions, your organization can unlock invaluable insights from vast troves of data, empowering you to make informed decisions, optimize processes, and drive continuous improvement.
Related Resources

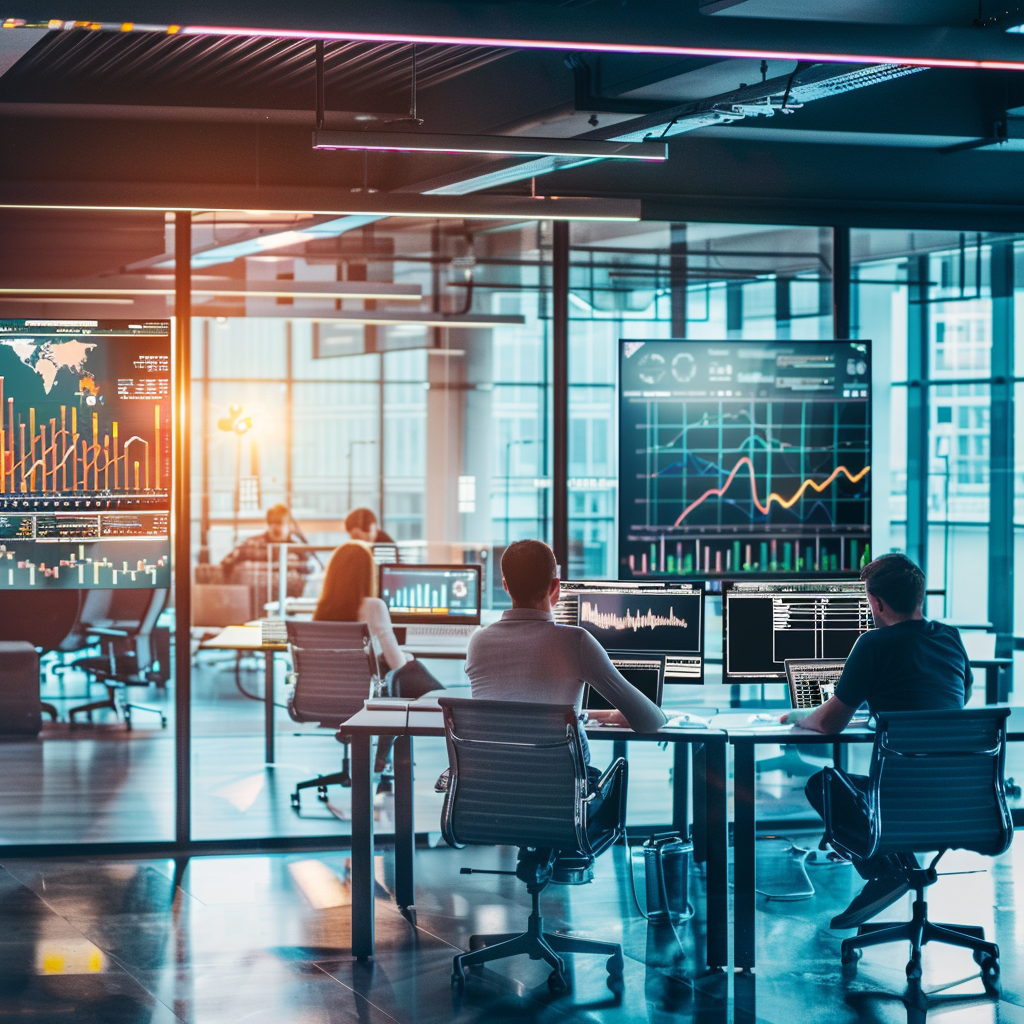
